Current Research Excerpts
March 27, 2023 2023-04-13 1:33Current Research Excerpts
Analysis of Genomic Variation
Each of our body cells carries a complete copy of our DNA and thus our complete genetic material, also known as the genome. The genome is organized into chromosomes: We inherit 23 chromosomes each from our mother and father. A chromosome consists of a very long DNA molecule, which is stabilized and spatially arranged by special proteins. The DNA encodes genetic information as a sequence of four different bases: Adenine (A), Cytosine (C), Guanine (G) and Thymine (T). In this sense, a chromosome can be simplified as a sequence of the letters A, C, G, and T. Genome research is therefore concerned with the question of how our genome sequence influences individual characteristics, i.e. what causal relationships exist between genetic variation and the expression of various characteristics. Many different traits can be of interest here: body size, susceptibility to a certain disease or intolerance to a certain drug.
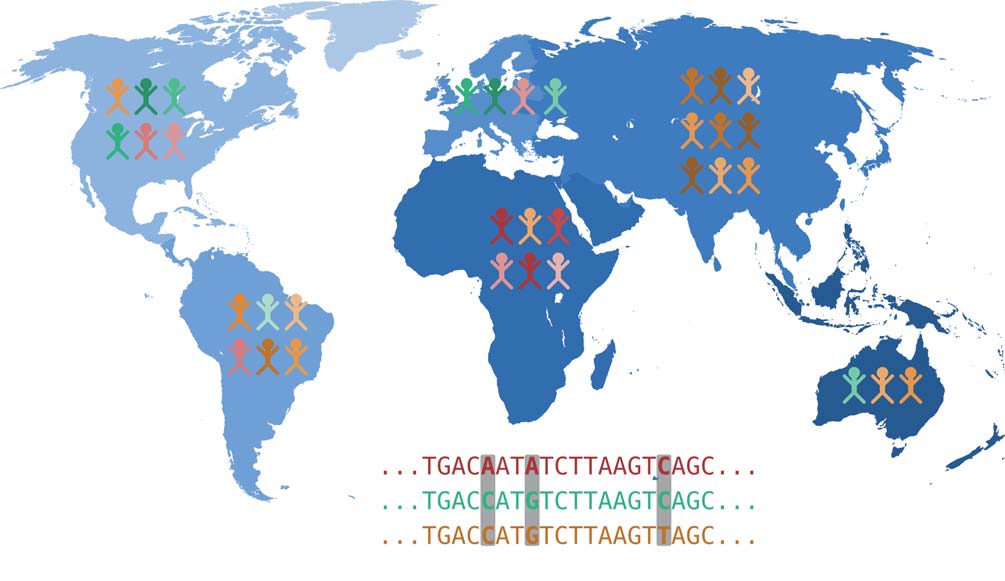
Genetic changes that are the cause of certain traits are mostly located in genes. These are DNA regions that serve as blueprints for proteins – or they are located in regions that control how much of a known protein is produced. From the genes encoded in the DNA, which can be thought of as blueprints as mentioned above, proteins are made in two steps. First, copies of these DNA segments are made, which are then available as RNA molecules. This process is called transcription. Then, proteins are produced from the RNA molecules, which perform a variety of functions. Thus, changes in DNA affect the proteins produced via the RNA detour. These changes can have considerable effects on the expression of traits and can, for example, be the cause of a genetic disease.
But how can differences in DNA or RNA be measured? In both cases, state-of-the-art sequencing methods (next generation sequencing, NGS), which are constantly being developed, can be used. The analysis of the huge amounts of data generated during sequencing poses challenges to scientists in the fields of computer science, mathematics and statistics. Researchers at the Center for Bioinformatics meet these challenges and have contributed significantly to the further development of modern analysis methods of DNA as well as RNA sequencing data.
Statistical Learning in Bioinformatics
In many areas of life, there has been an exponential growth of data in recent years. On the one hand, this is due to increasingly powerful computers that are able to process and store large amounts of data, but on the other hand, it is also due to new measurement methods in many areas of science, the so-called high-throughput methods. This can be seen, for example, in physics in measurements carried out at CERN, but also in biology there are ever more rapidly increasing amounts of data (e.g. genome, proteome and epigenome data). This flood of data makes it impossible to infer fundamental relationships in the data by just looking at them. For example, with genome sequences, we want to know where genes are located. If we can find out where genes are located through additional experiments, we can use statistical learning techniques to learn general sequence properties of genes, which can then be used in a predictive model. This makes it possible to predict the positions of genes for additional genome sequences. This approach is particularly interesting when the additional experiments are time-consuming and expensive, which is true for many areas of biology.
The methods used in this scenario belong to the group of statistical learning methods. However, they are also applied and developed in other fields, such as filtering out spam emails, predicting the most appropriate advertisements, or predicting stock trends. In bioinformatics, statistical learning is present in all important areas.
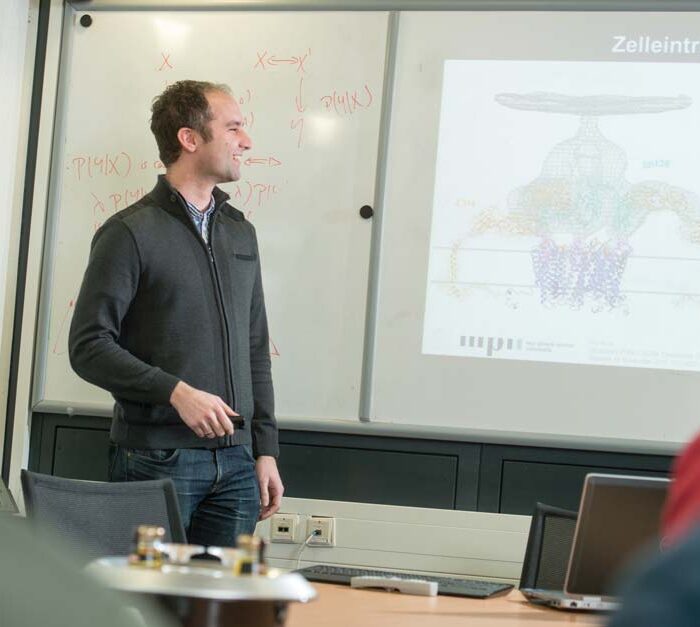
Statistical learning also occupies an important place at the Center for Bioinformatics. For example, Prof. Lengauer’s department has been developing new methods for predicting the resistance of the HI virus to certain antiviral drugs for more than a decade. The servers that make these methods freely available are used worldwide.
Another important area of biology is the study of evolutionary relationships between different species. Here, phylogenetic trees, also called phylogenetic trees, are determined. There, the distance in the tree indicates how far organisms are from each other in evolutionary terms. At ZBI, methods have been developed that significantly improve the learning of these phylogenetic trees.
Also of high importance is the field of cancer data analysis. Here it is interesting to find specific subgroups of patients using unsupervised learning methods, but also to find out which changes in the regulation of proteins have an impact on the severity of cancer. In this area, ZBI has developed new methods that, for example, allow us to combine measurements of different biological properties to generate an easily understandable representation of the data and find groupings based on them. In addition, we have also looked at cases where measurements for different patients are not entirely comparable and developed learning methods that can compensate for this effect.
Biological Networks
The metabolism of all living organisms is based on complex biochemical interactions and reactions of hundreds of thousands of molecules, including proteins, DNA and RNA molecules, lipids, and sugar molecules. A central role as information carriers is played by the genomes, and in particular by certain sections in the genomes called genes, which store the information for the synthesis of proteins and non-protein-coding RNA molecules.
Thanks to rapid scientific advances in biotechnology and bioinformatics, our knowledge of metabolic processes has increased so much in recent decades that the processing and storage of this knowledge alone represents a major challenge: It can now only be mastered by means of powerful bioinformatics databases. The corresponding data also form the basis for simulations of metabolic processes, which not only provide new insights into natural biological processes, but also into the causes of diseases and pathogenic mechanisms. These insights can in turn lead to new diagnostic and therapeutic approaches. In corresponding simulations, metabolic processes are usually modeled by means of graphs, which are also referred to as biological networks.
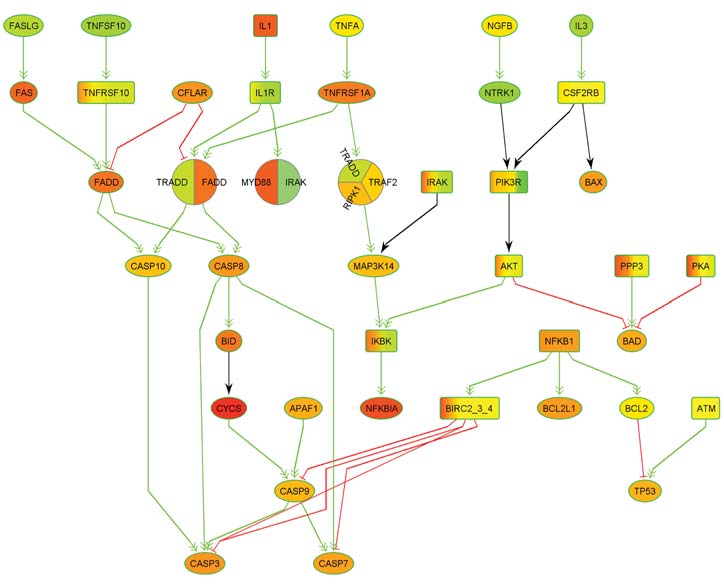
Here, the nodes of the networks represent the molecules involved and the edges represent the biochemical interactions between the molecules. In genetic diseases, the genetic aberrations present cause activity changes in networks that lead to disturbances in molecular information transfer. The modified components are also referred to as deregulated processes or networks. One focus of research at ZBI is the development of new methods for the detection of deregulated processes and subnetworks.
Biomolecular Interactions
Biomolecular interactions are the basis of all physical processes in living organisms. In fact, almost all biochemical reactions in living cells are based on molecular interactions between proteins, nucleic acids, sugars, lipids (fats) and low molecular weight ligands. Understanding these interactions is an indispensable basis for understanding the development of organisms and diseases, or susceptibility to pathogens. Bioinformatics can be used to generate detailed structural models of molecular complexes, energetically evaluate alternative positions of agents in the binding pocket of a protein, model binding processes, and also provide insight into the theoretical basis of such interactions.
Methods for studying biomolecular interactions are being developed at the Center for Bioinformatics.
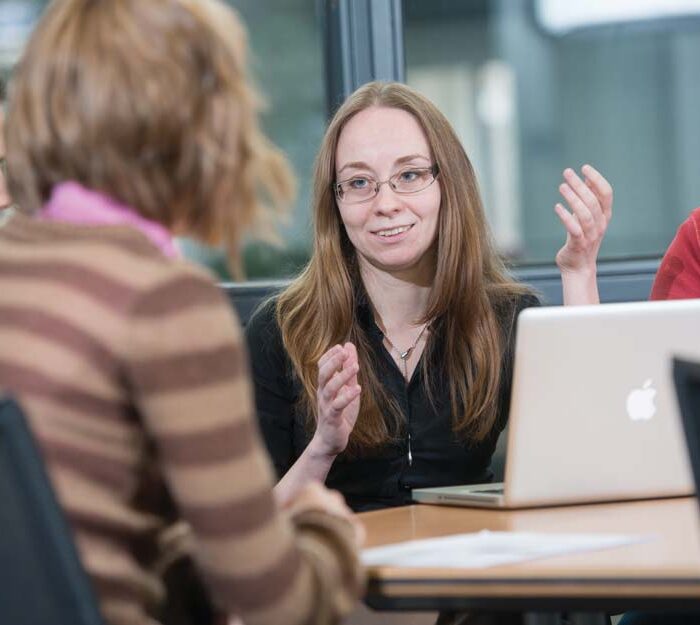
Epigenetics
Epigenetics is an area of research that is now the focus of worldwide attention. The reason for this is that after the sequencing of the human genome twelve years ago, it quickly became clear that essential aspects of cell regulation cannot be read directly from genomic DNA – after all, this is the same in all cells. In contrast, different cells differ considerably from one another – for example, cells in different tissues as well as diseased from healthy cells. Furthermore, central biological processes, such as the response to stress and aging, influence the cell state extensively. These factors of cell regulation stem from the dynamic organization of the genome in the cell nucleus, which in turn is based on chemical modifications of the genomic DNA and the surrounding molecular framework of proteins.
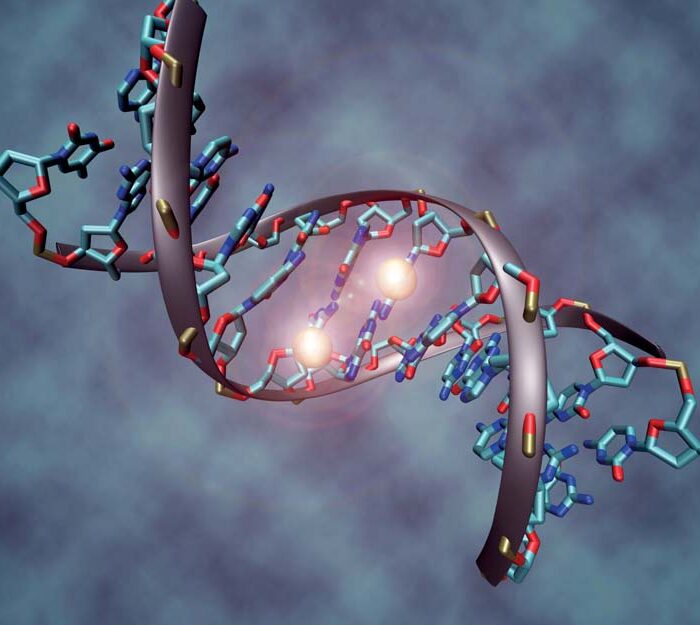
Epigenetics was initially defined in the 1980s as the science of inheritance of those traits that are not manifested by information encoded in DNA. However, it then quickly became apparent that such inheritance processes, as well as cell regulation in general, are based on the complex way in which the genome is packaged in the cell nucleus. Special proteins are involved in this packaging, which together with the genomic DNA form the so-called chromatin. The chromatin fulfills two tasks. First, the genomic DNA, which measures 2 m in its entire length, must be accommodated in the cell nucleus with a radius of one thousandth of a millimeter. Second, the parts of the genomic DNA in which information is encoded that is not needed by the cell must be protected from being read. Since the genomic in formation needed by the cell depends on the tissue and the cell state, chromatin is highly dynamic. Its structure is controlled by multiple chemical modifications of the DNA itself and its surrounding proteins. The totality of these modifications is referred to as the epigenome.
With molecular biology techniques developed in the last two decades, these modifications can be measured (epi-) genome-wide. Thus, the epigenome has become mappable. While a human has only one genome, in contrast, he has many epigenomes. In principle, epigenomes differ in all of the approximately 200 tissue types of the organism. In addition, they differ in healthy and pathologically altered cells in each cell type. Knowledge of the epigenome is considered a prerequisite for understanding the processes in the cell type in question. For this reason, the International Human Epigenome Consortium (IHEC) was founded a few years ago – a worldwide association of scientists with the goal of mapping at least 1,000 epigenomes of human cells in the next few years. The Center for Bioinformatics is involved in IHEC in two places (see projects DEEP and BLUEPRINT).
Infectious Diseases
Infectious diseases are a constant challenge for medical research. The reason is that fierce competition between different species has developed in nature since earliest times. This competition is still in full swing today. We humans become particularly aware of it through the activity of disease-causing pathogens. The pathogen seeks its advantage in infecting or colonizing the human host. The infected human organism defends itself against the pathogen with the help of its immune system and, in more recent times, supported by drug therapy. The pathogen in turn responds by adapting to the host. This adaptation does not take place primarily in the individual pathogen, but rather through an evolutionary change in the genome of the pathogen in subsequent generations. They thus produce characteristics that are favorable for survival in the host in the future. As a result of the constant change in the pathogen, new therapeutic strategies must then be developed again and again – a race is on between the pathogen and us humans as hosts.
Due to their high relevance for human health but also due to the exemplary evolutionary scenario they are based on, infectious diseases represent a rich fund of highly interesting and relevant research problems. The Center for Bioinformatics has chosen this area as one of its foci. We study infectious diseases from a variety of perspectives. However, the focus is on the evolutionary race between host and pathogen.
At the center, we study infectious diseases with particularly high evolutionary dynamics. The most dynamic of these is HIV, which has a high mutation rate and can therefore evolve rapidly in the body of any patient. At the Center, HIV is studied at various levels.
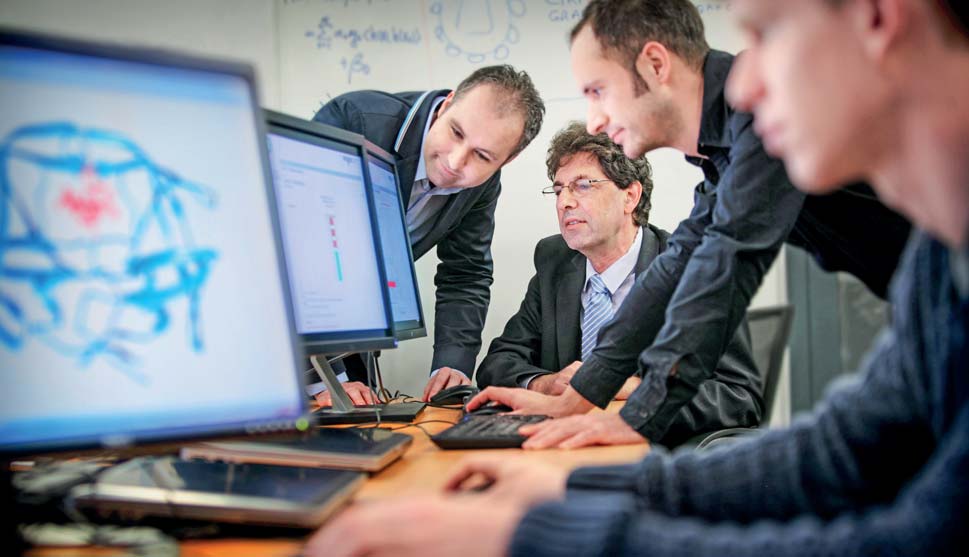
Compared to viruses, bacteria have a much more complex genome. This allows them to adapt to a host or to drug therapy in a more diverse way. For this reason, progress in the field of research on bacterial infections follows that on viral infections. Everyone is aware of the development of resistance in bacteria in the context of the declining effectiveness of antibiotics. At the Center, we study such resistance phenomena and exploit bacterial properties to develop new drugs. Finally, the center is researching a large-scale project that aims to transfer the already successful approach to bioinformatics analysis of viral resistance to bacteria.
Cancer
Despite the considerable progress made in oncology in recent decades, cancer is still responsible for 13% of all deaths worldwide, making it a leading cause of death directly after cardiovascular disease.
The causes of cancer are multifactorial. Genetic aberrations as well as environmental influences and lifestyle contribute to the development of cancer. Today, treatment is still largely based on surgery, radiation and chemotherapy. Although most patients respond to these treatments, not all are cured. A major reason for this is the heterogeneity of tumors, which is mainly due to “cellular evolution” occurring in tumors. This in turn is triggered by high rates of cell division and mutation and a failure of cellular repair mechanisms. In simplified terms, this means that a tumor is usually composed of genetically different tumor cells. Many of these cells can be killed by therapy, while other tumor cells are either already resistant to the therapy or can develop into resistant cells as a result of the appearance of new mutations.
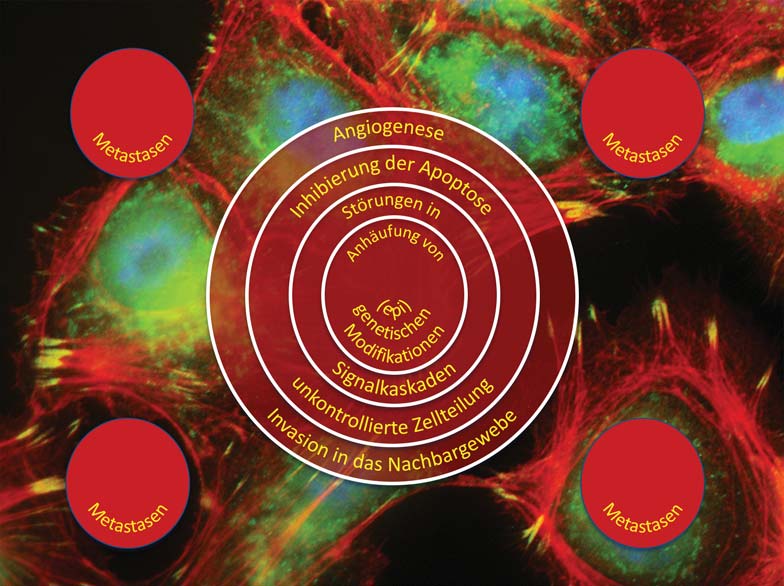
The chances of recovery from many types of cancer still depend heavily on the stage of development at which the disease is diagnosed. Here, the simple statement still applies: the earlier, the better. In addition to imaging methods, non-invasive diagnostic approaches based on biomarkers in body fluids play a central role in early detection. Research groups at ZBI have developed new diagnostic approaches based on high-dimensional profiles of tumor antigens and microRNAs for a number of cancers. These have a higher accuracy than the currently approved diagnostic methods. In addition to numerous publications, these projects have also resulted in a number of patents. For one method, the validation studies necessary for commercialization are already underway. To date, they have shown very promising results.